A Hybrid Approach to Movie Recommendation System

DOI:
https://doi.org/10.54060/a2zjournals.jmss.62Keywords:
Recommendation System, Content-Based Filtering, Collabora-tive Filtering, Hybrid recommendation systemAbstract
Recommendation Systems (RS) have become indispensable in today's digital ecosystem, influencing decisions and experiences across several platforms. This paper delves deeply on RS, including its history, functionality, and relevance. It covers many aspects of RS, such as Content-Based and Collaborative Filtering, using examples from a variety of industries, including e-commerce and entertainment. The paper also describes and covers empirical analysis methodologies for comparing RS efficacy and providing a framework. By conducting a qualitative and quantitative analysis, compared these three recommendation systems i.e. content based collaborative and Hybrid. This mixed analysis approach was necessary as Content-Based Filtering systems are not easily quantifiable, and for a movie recommendation system, the qualitative aspect holds significant importance. Through our analysis, it became evident that a hybrid recommendation system consistently outperforms standalone methods in terms of recommendation accuracy and relevance.
Downloads
References
V. M, and T. K, “History and overview of the recommender systems”, in Collaborative Filtering Using Data Mining and Analysis, V. Bhatnagar, Ed. Hershey: IGI Global, pp. 74-99, 2016.
M. Rouse, “What is collaborative filtering?”, WhatIs.com, para. 1, Aug. 11, 2017, [Online], Available: https://whatis.techtarget.com/definition/collaborative-filtering
C.S. Perone, “Machine Learning :: Cosine Similarity for Vector Space Models (Part III)”, Dec. 09, 2013, [Online], Available: http://blog.christianperone.com/2013/09/machine-learning-cosine-similarity-for-vector- space-models-part-iii/
B. Sarwar, G. Karypis, J. Konstan and R. John, “Item-based Collaborative Filtering recommendation algorithms”, 2001, pp. 285-295.
Z. Zhao, M. Shang, “User-based collaborative-filtering recommendation algorithms on Hadoop”, in Third International Conference on Knowledge Discovery and Data Mining, Phuket, Thailand, Jan. 09, 2010, IEEE, pp. 478-481.
R. V. Meteren, M. V. Someren, “Using Content-Based Filtering for recommendation”, 2000.
Y. Shih and D. Liu, “Hybrid recommendation approaches: Collaborative Filtering via valuable content information”, in Proceedings of the 38th Annual Hawaii International Conference on System Sciences, Big Island, USA, Jan. 06, 2005, IEEE, pp. 217b-217b.
G. Adomavicius and A. Tuzhilin, “Toward the next generation of recommender systems: A survey of the state-of-the-art and possible extensions”, in IEEE Transactions on Knowledge & Data Engineering, pp. 734-749, Apr. 25, 2005.
C. Ziegler, S. M. McNee, J. A. Konstan and G. Lausen, “Improving recommendation lists through topic diversification”, in Proceedings of the 14th international conference on World Wide Web, Chiba, Japan, ACM, pp. 22-32, May. 14, 2005.
S. Min and I. Han, “Detection of the customer time-variant pattern for improving recommender systems”, Expert systems with applications, vol. 28, no. 2, pp. 189-199., Nov. 2004
H. Lieberman, “Letizia: An Agent That Assists Web Browsing”, IJCAI, pp. 924-929, 1995.
B. Mobasher, “Recommender Systems,” Kunstliche Intelligenz, Special Issue on Web Mining, BottcherIT Verlag, Bremen, Germany, pp. 41-43, 2007.
M. Göksedef and S. G. Öğüdücü, “Combination of Web page recommender systems”, Expert systems with applications, vol. 37, no. 4, pp. 2911-2922., Apr 2010.
M. Y. H. Al-Shamri and K. K. Bharadwaj, “Fuzzy-genetic approach to recommender systems based on a novel hybrid user model”, Expert systems with applications, vol. 35, no. 3, pp.1386-1399., Oct. 2008.
F. M. Harper and J.A. Konstan, “The MovieLens Datasets:History and Context”, ACM Transactions on Interactive Intelligent Systems (TiiS) - Regular Articles and Special issue on New Directions in Eye Gaze for Interactive Intelligent Systems (Part 1 of 2), Article 19, vol. 5, no. 4, pp. 12-14., Jan. 2016
P. Singh, G. Srivastava, S, Singh, & S. Kumar, “Intelligent movie recommender framework based on content-based & col-laborative filtering assisted with sentiment analysis”, International Journal of Advanced Research in Computer Science, vol. 14 no. 3, 2023.
B. Hazela, P. Asthana, S. Singh, and G. Srivastava, “Enhance Movie Recommender System Using Machine Learning Tech-niques”, Proceedings of the Advancement in Electronics & Communication Engineering, 2022, Available at SSRN: https://ssrn.com/abstract=4159215 or http://dx.doi.org/10.2139/ssrn.4159215
G. Srivastava, V. Singh, S. Kumar, “Opinion Mining-Assisted Intelligent Program Selection Employing Fuzzy SWARA Mecha-nism”. In: Tanwar, S., Wierzchon, S.T., Singh, P.K., Ganzha, M., Epiphaniou, G. (eds) Proceedings of Fourth International Conference on Computing, Communications, and Cyber-Security. CCCS 2022. Lecture Notes in Networks and Systems, vol 664, 2023. Springer, Singapore. https://doi.org/10.1007/978-981-99-1479-1_45.
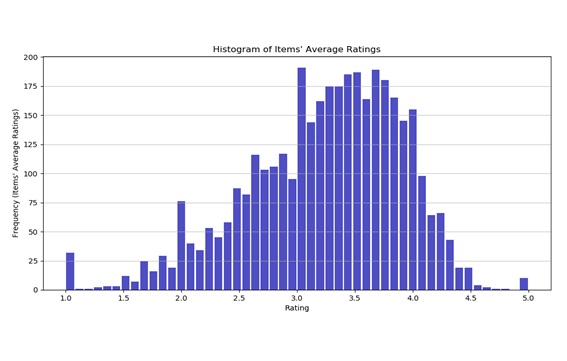
Downloads
Published
How to Cite
CITATION COUNT
Issue
Section
License
Copyright (c) 2024 Abhay Yadav, Garima Srivastava, Dr. Sachin Kumar

This work is licensed under a Creative Commons Attribution 4.0 International License.