Improving the Utility of Web Surfing Using AI Techniques

DOI:
https://doi.org/10.54060/jmss.v3i1.43Keywords:
Artificial intelligence, web browsing, machine learningcontent filtering, collaborative filteringAbstract
This paper aims to improve web surfing security and utility by leveraging artificial intelligence (AI) and machine learning (ML) algorithms. A more personalized, efficient, and secure online experience is achieved through improving the utility and security of web browsing. Web browsing has become an integral part of our daily lives in the digital age. Users often face information overload, irrelevant content, security threats, and privacy concerns. AI and machine learning are used in the proposed system to refine web browsing. A web browsing utility that uses user preferences, interests, and browsing behavior to provide personalized recommendations, filter out irrelevant content, and enhance overall utility is the objective of this paper.
Downloads
References
J. Wang, J. Liu, and Y. Liu, "Collaborative filtering with matrix factorization for personalized recommendations," in Proceedings of the ACM Conference on Recommender Systems, pp. 123-136, 2018.
P. Melville, R. J. Mooney, and R. Nagarajan, "Content-based book recommending using learning for text categorization," in Proceedings of the ACM Conference on Recommender Systems, pp. 195-202, 2016.
J. Lin and D. M. Blei, "Collaborative filtering for implicit feedback datasets," in ACM Transactions on Intelligent Systems and Technology, vol. 5, no. 4, pp. 1-34, 2014.
M. Deshpande and G. Karypis, "Item-based top-N recommendation algorithms," in ACM Transactions on Information Systems, vol. 22, no. 1, pp. 143-177, 2004.
L. Ungar and D. Foster, "Clustering methods for collaborative filtering," in ACM Transactions on Information Systems, vol. 22, no. 2, pp. 231-262, 2004.
S. Zhao, L. Huang, and Q. He, "Personalized recommendation algorithm based on user behavior and content analysis," in Proceedings of the IEEE International Conference on Data Mining, pp. 654-661, 2019.
R. Herbrich, T. Graepel, and K. Obermayer, "Large margin rank boundaries for ordinal regression," in Advances in Large Margin Classifiers, pp. 115-132, 2000.
J. S. Breese, D. Heckerman, and C. Kadie, "Empirical analysis of predictive algorithms for collaborative filtering," in Proceedings of the Fourteenth Conference on Uncertainty in Artificial Intelligence, pp. 43-52, 1998.
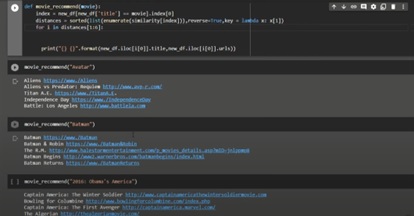
Downloads
Published
How to Cite
CITATION COUNT
Issue
Section
License
Copyright (c) 2023 Harsh Deep Keshari, Dr. Sheenu Rizvi

This work is licensed under a Creative Commons Attribution 4.0 International License.