Attrition Unveiled: Analyzing Trends and Strategies in Employee Turnover

DOI:
https://doi.org/10.54060/a2zjournals.jmss.72Keywords:
Employee attrition, EDA, Retention strategies, data visualisation, Python, TurnoverAbstract
This study performs an Exploratory Data Analysis (EDA) on employee attrition data using Python to identify key factors influencing turnover. Employee attrition affects organizational performance, making it crucial to understand its root causes for effective retention strategies. The analysis considers various employee attributes, including demographics (age, gender) and job-related factors (salary, satisfaction, tenure). The process involves data cleaning, followed by univariate, bivariate, and multivariate analyses to explore variable relationships. Visualizations such as bar charts, scatter plots, and heatmaps aid in identifying patterns and high-risk employees. The findings offer actionable recommendations to enhance retention and serve as a foundation for an organization to make data-driven decisions.
Downloads
References
H. H. Nguyen and T. Raveendran, "A study on employee attrition using predictive modelling techniques," Journal of Hu-man Resource Management, vol. 6, no. 4, pp. 121-129, 2017.
L. W. Porter and R. M. Steers, "Organizational, work, and personal factors in employee turnover and absenteeism," Psy-chological Bulletin, vol. 80, no. 2, pp. 151-176, 1973.
R. Saeed and M. Faheem, "A comprehensive review of factors influencing employee attrition using data analytics," Journal of Business Analytics, vol. 5, no. 2, pp. 213-227, 2020.
H. Kaur and K. Singh, "Data Mining approaches for predicting employee attrition," IEEE Transactions on Computational Social Systems, vol. 8, no. 3, pp. 458-465, 2021.
A. Singh and A. Verma, "Exploring the role of organizational culture in employee retention: A data-driven approach," Management Research Review, vol. 45, no. 5, pp. 800-815, 2022.
H. Tang and X. Li, "Predictive analytics for employee turnover in the banking sector," Journal of Business Intelligence, vol. 10, no. 3, pp. 62-73, 2018.
Z. Zhang and Y. Zheng, "Understanding employee turnover intention: A deep learning approach," Journal of Human Re-source and Sustainability Studies, vol. 8, no. 2, pp. 65-72, 2020.
M. Suresh and J. Vardhan, "A study on factors influencing employee attrition using logistic regression," Journal of Busi-ness and Management, vol. 20, no. 3, pp. 50-58, 2018.
R. Ali and M. Mehdi, "Employee turnover prediction using machine learning techniques," Journal of Artificial Intelligence Research & Development, vol. 34, no. 1, pp. 45-53, 2019.
C. Hsieh and S. Lewis, "Data-driven analysis of factors influencing employee retention: A machine learning approach," International Journal of Advanced Computer Science and Applications, vol. 10, no. 4, pp. 135-142, 2019.
M. Z. Hussain, T. B. Alam, and R. Singh, "Understanding employee turnover patterns through data analytics: A case study approach," in Proc. IEEE Int. Conf. Big Data Analytics (BDA), Bengaluru, India, 2020, pp. 65-72.
A. Hasan and S. Sharma, "Predicting employee attrition using machine learning techniques: A case study," in Proc. IEEE Int. Conf. Advances in Big Data, Computing and Data Communication Systems (icABCD), Durban, South Africa, 2018, pp. 150-155.
A. S. Deshpande, "Employee turnover prediction using machine learning techniques: A case study," in Proc. IEEE Int. Conf. Data Mining (ICDM), Dallas, TX, USA, 2017, pp. 298-305.
S. Mallik and R. Sinha, "An analysis of employee attrition using predictive models," in Proc. IEEE Int. Conf. Computational Science and Engineering (CSE), Singapore, 2019, pp. 105-110.
C. Hsieh and S. Lewis, "Data-driven analysis of factors influencing employee retention: A machine learning approach," International Journal of Advanced Computer Science and Applications, vol. 10, no. 4, pp. 135-142, 2019.
J. Han, M. Kamber, and J. Pei, Data mining: Concepts and techniques, 3rd ed., Morgan Kaufmann, 2011.
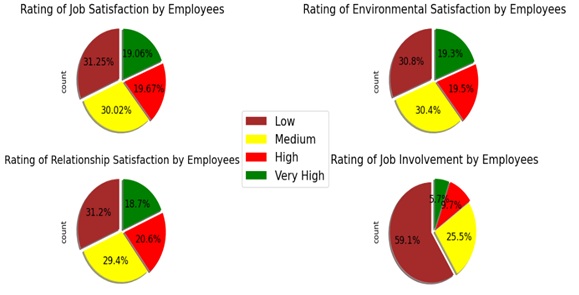
Downloads
Published
How to Cite
CITATION COUNT
Issue
Section
License
Copyright (c) 2024 Aman Shrivastava, Dr Pooja Khanna

This work is licensed under a Creative Commons Attribution 4.0 International License.