Human Activity Tracker and Recognition

DOI:
https://doi.org/10.54060/jmss.2023.44Keywords:
Deep Learning, Machine Learning, Artificial Intelligence, Human Activity Recognition, Feature ExtractionAbstract
Human Activity Recognition (or, HAR) is a piece of software that uses AI algorithms to recognize and categories human physical activity. By analyzing signal data from multiple sensors such as accelerometers, gyroscopes, and magnetometers, the sys-tem is meant to recognize and categorize physical activities such as walking, running, leaping, ascending stairs, and others. To recognize human activity patterns, the HAR system employs signal preprocessing, feature extraction, and classification algo-rithms. The use of simulated intelligence techniques such as deep learning computa-tions, convolutional brain organizations, and supporting vector machines has im-proved the display of HAR frameworks. The system may be utilized for a variety of purposes, including security, sports, fitness, and healthcare. In general, the HAR framework provides a beneficial value to robotized human activities. Man-made reasoning (Artificial Intelligence) plays an important role in Human Activity Recogni-tion by allowing frameworks to learn and adapt to new conditions. In general, HAR framework is beneficial asset to robotized human movement recognition, working with the advancement of clever frameworks that can research human be-haviour and work on personal fulfilment. Overall, Human Activity Recognition Using Computerized Reasoning is promising innovation that enables intelligent frame-works to perceive and group human activities gradually. This breakthrough has the potential to disrupt several businesses and improve people's personal pleasure by enabling personalized medical treatment, improving game execution, and improving street safety. The creation of this software sets the path for more study into themes such as the relationship between individual health status and physical activity. Over-all, creating a fruitful Human Action Acknowledgement project utilizing recordings necessitates a broad understanding of AI and Profound Learning methods. As a re-sult, success of this project highlights the value of creativity and perseverance in learning. Finally, it is the initial step towards developing more advanced systems that will improve people's lives in the future.
Downloads
References
J. Wang, Y. Chen, H. Hao, and B. Peng, “A Survey on Human Activity Recognition Using Wearable Sensors,” IEEE Access, vol. 7, pp. 37530–37549, 2019. https://doi.org/10.1109/access.2019.2903901
J. Gao, Z. Yang, J. Wu, & J. Zhang, “A comprehensive review of human activity recognition with wearable sensors,” Sensors, vol. 20, issue 4, pp. 1238. https://doi.org/10.3390/s20041238
S. Ranawaya and P. K. Atrey, “Human activity recognition using machine learning techniques: A review,” Journal of Ambient Intelligence and Humanized Computing, vol. 10, no. 4, pp. 1271–1287, 2019. https://doi.org/10.1007/s12652-018-0895-8
L. Atallah, B. Lo, and R. King, “Human activity recognition using a single accelerometer placed at the wrist or ankle,” Medicine and Science in Sports and Exercise, vol. 50, no. 3, pp. 624–633, 2018. https://doi.org/10.1249/mss.0000000000001471
W. A. Khan, & K. Y. Lee “Human activity recognition using smartphone sensors and deep learning,” Sensors, vol. 18, no. 5, 2018. https://doi.org/10.3390/s18051499
F. Harrou and C. Tanougast, “Human activity recognition using deep learning: A review,” IET Computer Vision, vol. 13, no. 5, pp. 452–460, 2019.. https://doi.org/10.1049/iet-cvi.2018.5467
A. Bulling, U. Blanke, and B. Schiele, “A tutorial on human activity recognition using body-worn inertial sensors,” ACM Comput. Surv., vol. 46, no. 3, pp. 1–33, 2014. https://doi.org/10.1145/2499621.2499652
S. J. Pan and Q. Yang, “A survey on transfer learning,” IEEE Trans. Knowl. Data Eng., vol. 22, no. 10, pp. 1345–1359, 2010. https://doi.org/10.1109/tkde.2009.191
M. Sharma, & B. R. Pachori, “Human activity recognition from accelerometer data using deep learning”. PloS One, vol. 11, no. 12, e0168700. https://doi.org/10.1371/journal.pone.0168700
W. Zhang, H. Li, D. Zheng, A. K. Sabbir, D. Xie, and D. Essam, “Human activity recognition using machine learning and deep learning techniques: A review,” Wireless Communications and Mobile Computing, 2020. https://doi.org/10.1155/2020/7015086
A. Tyagi, P. Singh, and H. Dev, “Proposed spatio‐temporal features for human activity classification using ensemble classification model,” Concurr. Comput., vol. 35, no. 6, pp. 1–1, 2023.
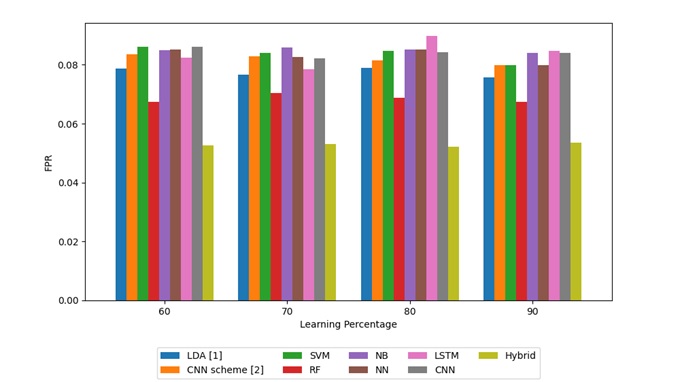
Downloads
Published
How to Cite
CITATION COUNT
Issue
Section
License
Copyright (c) 2023 Vishisht Saxena, P. Singh, Avimanyou Vatsa

This work is licensed under a Creative Commons Attribution 4.0 International License.